General Overview of Retina Recognition
The human retina is a thin layer of tissue with intricate blood vessels located at the back of the eye. A part of face liveness detection, retinal recognition focuses on the patterns of these blood vessels which are reported to be highly unique and stable at the same time. Retinal vasculature — artery, vessels and arterioles — stay mostly unchanged throughout human life just like the brain structure. The only factors that can alter it are diseases like cataracts, diabetic retinopathy and some others.
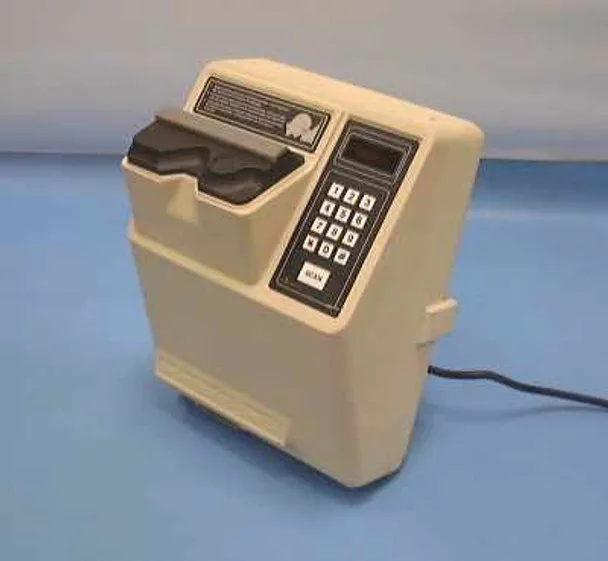
Apart from diagnosing health problems and even mortality risks, retinal scan can be used as for identifying an individual's identity with high accuracy as the blood vessel patterns are known to never repeat within the human population (not even between monozygotic twins). A certain disadvantage of this method is that it cannot be considered as a passive liveness technique. Initially, the method was proposed by Carleton Simon and Isadore Goldstein in 1935, although a similar theory was introduced earlier by Levinson, as well as Haber and Blaschek. The patent for a retina-scanning device was received in 1978 by the EyeDentity company. The concept was commercialized in 1984 when the company began making high-quality retinal scans. The machines used the principle of infrared radiation (IR), which allowed capturing the image of retinal blood vessels without altering pupil diameter. It is suggested that retinal recognition can play a major role in facial anti-spoofing and its various types, countermeasures and challenges.
Retinal Anatomy


Retina is the only component of the human Central Nervous System (CNS) that can be observed noninvasively. It is composed of light-sensitive cells with a 0.2–0.4 mm thickness, which capture light rays coming through the pupil and lens. Eye retina contains photoreceptors that are divided into cones (7 million) and rods (75–150 million).

While rods can detect light, they transmit grayscale visuals. As for cones, they are divided into red, green and blue groups, colorizing human vision. Other two vital elements of the retina include the optical disc (blind spot) and the yellow spot (macula). Optical disk has no photoreceptors, so it cannot provide visual signal. At the same time, macula with a 5-mm diameter has the highest quantity of photoreceptors, with the most number of cones — resulting in detailed and sharp vision.

In the center of the macula, fovea centralis is located, which is responsible for the direct view. Macula is not actually yellow during a person’s life even though it contains lutein observed in egg yolks. It attains the yellowish color tone only in case of Age-Related Macular Degeneration (AMD) or due to the postmortem changes.

General Structure of Retina Recognition Systems
Currently, retinal recognition is challenging as it requires specific equipment and a high degree of cooperation from the user. Typically, the procedure requires:
- Eye positioning. The user must put their eye very close to the lens of the retinal scanner.
- Gazing. The user should unremittingly gaze into the lens, while remaining perfectly still.
- Focusing. The user should also focus on the green light for 1 minute, while the scan is taking place, which is somewhat uncomfortable.
Due to the procedural difficulty, retinal recognition is sparingly seen in public places and only used at high-security sites and facilities. At the same time, due to the arduousness of the process, risk of a retina being fabricated is minimal. Apart from image capturing, the procedure also includes pre-processing, normalization, image enhancement, feature extraction, and other steps vital for successful identification.
Devices for Getting Retina Information
One of the simplest tools for retrieving retinal data is an ophthalmoscope, which includes a light source and a semi-pervious mirror or a mirror with a hole located in the observation axis (at a 45° angle). However, the method requires a certain skill level and provides a limited investigation area, which may be somewhat in dissonance with the liveness certification standards.

Fundus photography is a more reliable and accurate approach. Based on indirect ophthalmoscopy, it employs a white light source to illuminate retinal area and a Charge-Coupled Device (CCD) sensor for scanning. As a result, images of the posterior segment of the optic nerve, yellow spots and the peripheral part of the retina can be obtained.


Retina Databases
A number of public and restricted retina databases are available. The publicly available examples include High-Resolution Fundus (HRF) dataset, Retina Identification Database (RIDB), E-ophtha, PAPILA, DRIVE, and Messidor and Messidor-2 (the last two are mostly used for medical research).

Vascular & Nonvascular Approaches
Retinal recognition has two vital methods: vascular and nonvascular.
Vascular approach
Vascular-based methods are implemented in many ways. One algorithm by Patwari et al. extracts blood vessels and bifurcation points. It employs Histogram equalization for image enhancement, 2-D median filtering and morphological operations. Another method dubbed RPRA relies on intersection points, maximum principal curvature of the Hessian matrix used for segmentation, thresholding and thinning algorithms, etc. Other approaches use Wavelet decomposition, edge location refinement, Frangi vessel enhancement, supervised classification of vessel outlines, 2D Gabor filtering, etc.

Nonvascular approaches
Nonvascular methods pay attention to other features of retinal images, such as statistics. A methods by Rehman et al. involves stages such as background removal, local contrast enhancement to pinpoint region of interest (ROI), Hue Saturation Value color space extraction, Gray-Level Co-Occurrence Matrix (GLCM), Euclidean distance-based classifier, etc. Another technique applies Fourier transform, while partitioning an image into a number of several half circles, which share the same center. Plus, Fourier Energy Feature (FEF) feature vectors are measured and matched with the Manhattan Distance against the registered image vectors.
Retina Recognition Methods Based on Neural Networks
A multilayer feed-forward neural network with the sigmoid activation function in output and hidden layers is suggested for retina recognition. The method employs vascular segmentation, feature vector extraction, -training set for 10,000 epochs, etc. Interestingly, the retinal images are made grayscale: this strategy allows economizing resources (less data for each pixel), while such images provide equal intensity in RGB space.

Another research suggests a Convolutional Neural Network (CNN) that can recognize unique parameters of the retinal vasculature. It includes such libraries as Keras high-level neural networks API and Tensorflow as backend engines. The method implies that fundus RGB photos should be transformed into green channel images due to their intensity values of light and dark being in fine balance.
Biological Effect of Infrared Radiation Exposure in Retina Recognition Systems
Currently, biometric systems employ IR-A radiation, which is in the 760-1400 nm frequency range. Generally, infrared radiation (IR) is not classified as ionizing. A human iris absorbs up to 98% of the IR light within a spectrum of 750 to 900 nm. Even though IR-A is seen as mostly harmless, IR radiation can still produce thermal damage to the human eye, including retina. In the long run, this can trigger miosis, delayed cataract, and other maladies.
Combining Retina & Iris Recognition
A multimodal system that comprises both iris and retina recognition provides greater accuracy and is more fail-proof in terms of recognition and anti-spoofing. A score level fusion of distances of both irises produces a 96.4% accuracy rate, while blood vasculature segmentation and other steps provide a 96.3% accuracy rate. The latter requires lesser storage capacity as only intersection points from the retinal vessel structure are needed.
FAQ
How to get biometric retinal data for person identification?
Retinal data is, by far, the hardest to obtain for personal identification.
Retina recognition is considered as highly unique and reliable outperforming even fingerprint modality. At the same time, it’s quite challenging to deploy it due to a number of reasons. First, it’s difficult to capture retinal data because of the eye anatomy. To do that, specialized equipment — fundus photography and ophthalmoscopes — should be used.
Second, retinal recognition and liveness detection demand cooperation from a person, making it an active liveness technique to an extent. Typically, a procedure includes three stages: eye positioning, gazing and focusing. Therefore, it becomes intrusive and requires more processing time unlike facial or voice recognition, which respond instantly.
What are the main retina datasets?
A select number of retinal datasets are proposed.
While retina images are harder to capture than face or fingerprints, there’s a selection of them as retinal photography is used in medicine for diagnosing ocular (and some other) diseases. Messidor and Messidor-2 datasets are two well-known examples assembled at the research center Mines Paris for diagnosing diabetic retinopathy. They provided paired fundus images supplied with third-party annotations.
Retina Identification Database (RIDB) was created with a TOPCON-TRC camera and offers 100 samples from 20 people. PAPILA is a public funds dataset with segmentations of the cup and optic disc. All can be used for retinal anti-spoofing as well.
What is the main advantage of retinal biometrics?
Human retina has unquestionable advantages in terms of anti-spoofing.
As suggested, retina recognition has a number of advantages that make it stand out in comparison to other modalities. First, it’s not as exposed as other biometric traits: fingerprints, face, iris, etc. Retina is an innermost layer of light-sensitive tissue, which is located at the back of an eye near the optic nerve. Due to such anatomical structure, retina can’t be observed with a naked eye or captured with a regular camera.
Specialized equipment used for fundus photography is required to retrieve retinal images. At the same time, it makes the procedure lengthy, cumbersome and costly when compared to other biometric and anti-spofing modalities. However, one of the major issues accompanying retinal recognition is that it might be sabotaged by obfuscation attacks.
References
- Retina Recognition Using Crossings and Bifurcations
- Retinal diseases
- EyeDentify 7.5 — the first commercial retinal scanner released in the 1980s
- Retinal age gap as a predictive biomarker for mortality risk
- Science: Eye Prints
- Retina Recognition Using Crossings and Bifurcations
- The Retina: Where Vision Begins
- Blind spot
- The Function of the Normal Macula
- Retinal Layers
- Fovea Centralis
- What is Lutein? By Allison Webster, PhD, RD
- Age-Related Macular Degeneration (AMD)
- Fovea centralis by Wikipedia
- Ophthalmoscope can be used for a simple retinal examination
- Color Fundus Photography
- White Light Sources
- Retinal scan by Wikipedia
- Advances in Computer Vision and Pattern Recognition
- High-Resolution Fundus (HRF) Image Database
- Retina Identification Database (RIDB)
- E-ophtha
- PAPILA
- DRIVE (Digital Retinal Images for Vessel Extraction)
- Messidor
- Messidor-2
- PAPILA: Dataset with fundus images and clinical data of both eyes of the same patient for glaucoma assessment
- Person identification using vascular and non-vascular retinal features
- An efficient retina pattern recognition algorithm (RPRA) towards human identification
- A Gentle Introduction To Hessian Matrices by Mehreen Saeed
- Bifurcation points in retinal vessels
- Retinal Identification
- A human identification system based on retinal image processing using partitioned fourier spectrum
- Feedforward neural network by Wikipedia
- Biometric retina identification based on neural network
- Image processing, pattern recognition: retinal biometric identification using convolutional neural network
- Keras
- Tensorflow
- Eye Safety Related to Near Infrared Radiation Exposure to Biometric Devices
- What Is Miosis?
- A robust person authentication system based on score level fusion of left and right irises and retinal features